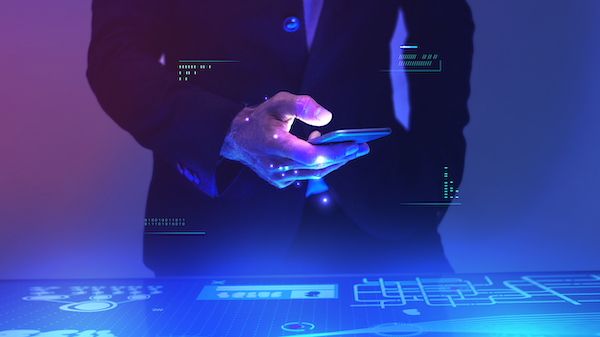
Productivity has always been a core goal for finance teams, investing in both enterprise resource planning (ERP) and integrated best-of-breed platforms to unlock efficiencies that enable strategic decision-making and transformation projects. For the finance organization, productivity can translate into a variety of value-added outcomes: more efficient cash application and reconciliations, shortened close cycles, executing early payment discount programs, and receivables programs to unlock trapped cash, to name a few examples. The CIO and CFO, as the delivery agents for automation and business intelligence, become a stronger and more valued partner for the overall finance organization by delivering automation and efficiency.
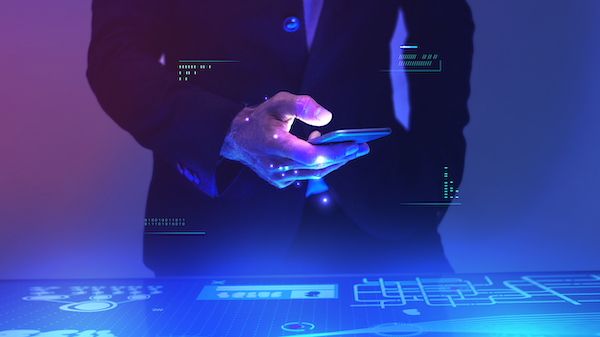
Recently, the migration to cloud ERPs has offered open API innovation providing integration via real-time API-based connectors between the ERP and a variety of external (e.g., accounts receivable (AR), accounts payable (AP), treasury, investment/FX portals and banks) and internal (e.g., business intelligence apps and data lakes) systems. This not only increases the speed of data transfer but also introduces additional time-saving features such as better confidence in wire/deal settlements, liquidity availability, and payment fraud detection across the ERP and ancillary systems. The increase in access to analytically focused apps is a win on its own, but when coupled with a networked ERP, CFOs are given a powerful solution to drive analytics-based decisioning. Automation, therefore, is a prerequisite to a higher order of analytics and business intelligence.
Augmented Analytics
A critical signpost on the road to business intelligence is the progression from a system of record to a system of insight. Today’s ERPs make for fantastic systems of record. As the single source of truth for financial operations, the modern ERP is accessible across the organization and designed to collect data and drive business workflow.
To become truly insightful, CIOs must provide their finance stakeholders with new, emergent technologies to ensure data becomes information that is structured and intelligent. CIOs – to keep their organizations competitive –are looking to artificial intelligence and machine learning, offering improved predictability, exception identification, or ideally both. This requirement for augmented analytics such as machine learning, a part of the AI family, has been especially fueled by the immense volume of data that finance teams have access to and are required to find meaning in.
One example includes B2B payments, managed by accounts payable (AP) within the CFO’s team. Payment workflows require multiple decision points, each necessitating a level of review by one or more individuals. Have there been enough approvals for this large dollar international payment, do we have the required documentation to approve the payment request, is this the correct bank account for our new vendor, and so forth. Individually these decisions and corresponding steps would be built within the AP workflow in the ERP and/or AP portal. However, in today’s world of payments fraud and real-time payments (which settle immediately and cannot be repatriated in the case of a mistake or fraud), a recommended practice by most CIOs and auditors is to perform an AP audit, a final screen of all outgoing payments to ensure compliance with internal payment policies and good corporate governance. Augmented analytics such as machine learning are critical for the task given such an audit cannot be performed over days, hours, or even minutes. It must be in real-time to ensure vendors are paid, payroll is funded, and financial contracts are settled with banks and counterparties. A machine learning algorithm can be trained on the entire payment history of the organization to identify payment anomalies – potentially suspicious payments that require further review before sending to banks for settlement.
Further analytics identify exceptions to payment policies, not only flagging non-compliant items but also learning from the false positives of prior analyses to ensure expediency and focus the CFO’s and internal audit’s time on truly serious items. Given the time restrictions as well as the increased data volumes, human eyes cannot keep up. The learned experience of artificially intelligent models is the only choice.
Another example involves the CFO’s need for precise and intelligent decisioning of enterprise liquidity, a responsibility which was especially on display at the height of the pandemic. Many CFOs struggled to deliver precise views into cash and liquidity, creating downstream challenges for payments and receivables teams on how to manage working capital. As a result, many supplier payments were delayed, and receivables unnecessarily (and expensively) accelerated to ensure sufficient liquidity was available to manage the business day to day. The predictability offered by artificial intelligence would have given treasurers, finance directors and their CFOs multiple views of the cash forecast across a variety of scenarios, the most alarming of which could have been modeled back to the 2008/2009 credit crisis. Finding meaning in historical data was a lifeline many CFOs did not have, which hindered data-driven, business intelligent decisions that could have saved organizations significant time and cost.
Achieving Business Intelligence
As CFOs look ahead to post-pandemic planning, they rely on their CIO partners to support their organizations through the combination of artificial intelligence and business intelligence tools. This marriage of analytics and insight, delivered by building out the ERP with a connected ecosystem of business applications, offers insightful data analyses presented in dynamic dashboards to simplify complex financial decision-making. Few things make a CFO happier than insightful information presented in one view, proactively offered and available on-demand. Armed with that perfect combination, CFOs can rest assured they have achieved business intelligence.